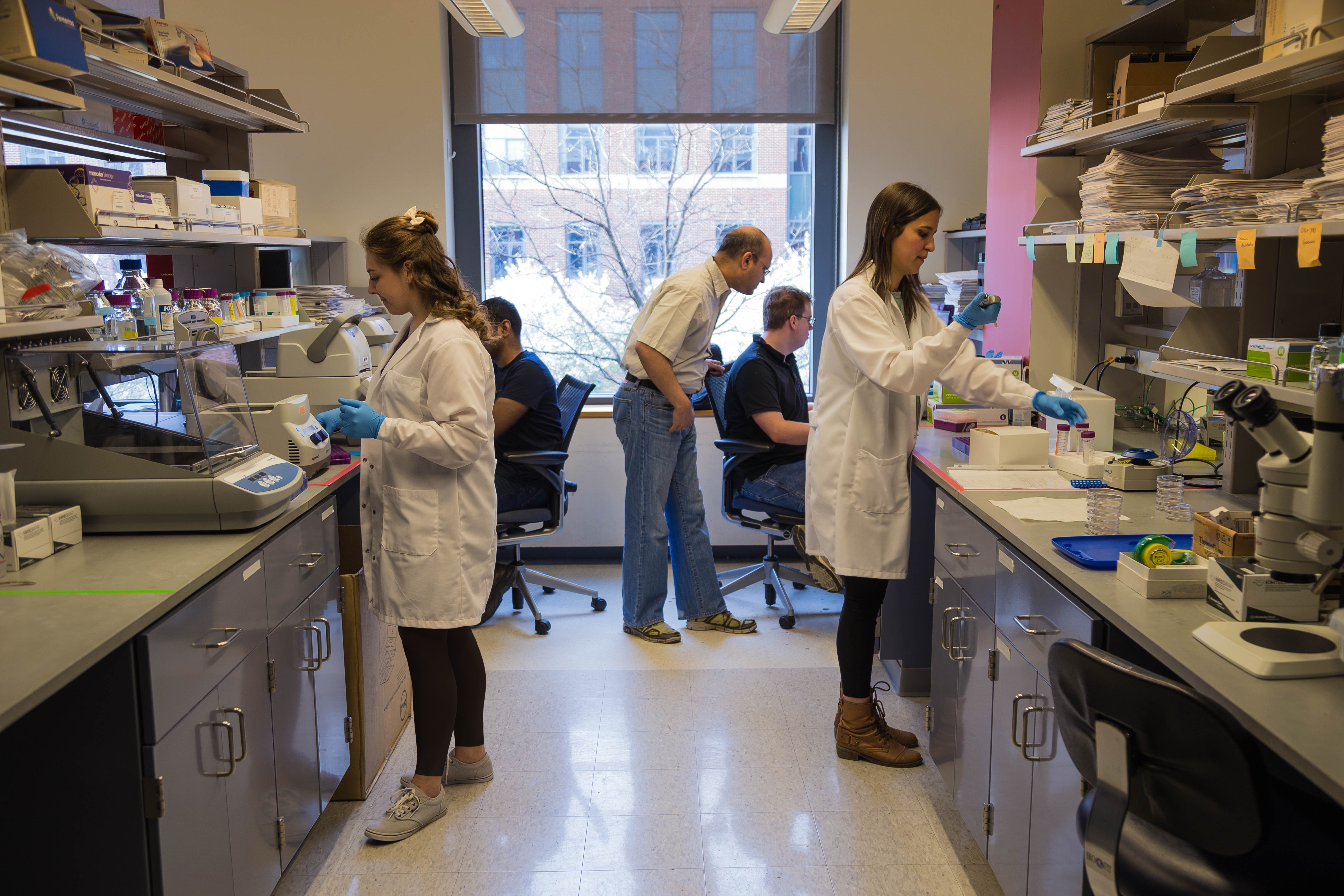
The primary focus of my research is to understand the genomic basis of phenotypic heterogeneity associated with neurodevelopmental disorders such as autism, schizophrenia, and intellectual disability, with a special focus on pathogenic copy-number variants (CNVs), or duplications and deletions in the genome.
As a graduate student in Sarah Elsea's lab, I found that individuals with Smith-Magenis syndrome (SMS) who carry a 17p11.2 deletion, encompassing the retinoic acid induced 1 (
RAI1) gene, have a typical constellation of phenotypes (
Girirajan et al, JMG, 2005). Using patient data and mouse models, we showed that haploinsufficiency of
RAI1 leads to a majority of SMS features, with little effect from other genes within the deletion region or in the genetic background (
Girirajan et al, GIM, 2006;
Girirajan et al, EJHG 2008;
Elsea and Girirajan, EJHG, 2008;
Girirajan et al, Mamm. Genome 2009;
Girirajan et al, EJMG 2009). As a postdoctoral fellow in Evan Eichler's lab, I was involved in identifying another class of CNVs associated with a wide range of neurodevelopmental outcomes and also reported in unaffected or mildly affected individuals (
Girirajan et al, PLOS Genet., 2011;
Cooper et al, Nat. Genet., 2011;
Girirajan et al, AJHG, 2013). While extensive phenotypic heterogeneity observed in individuals with these CNVs precluded the involvement of a single causative gene, as in SMS, we found that variants in the genetic background contribute to the observed variability (
Girirajan et al, Nat. Genet. 2010;
Girirajan and Eichler, HMG 2010;
Girirajan et al, NEJM, 2012).
As an independent investigator at Penn State, my research combines gene discovery and dissecting phenotypic heterogeneity using large-scale genomic studies (
Polyak et al, Genome Med. 2015;
Polyak et al, AJMG, 2015;
Pizzo et al, EJHG, 2016) with studying the molecular functions and mechanisms of CNV pathogenicity in model systems (
Iyer and Girirajan, Brief. Funct. Genomics, 2015) and developing algorithmic methods for genomic (
Wang et al, Sci. Rep., 2017) and functional data analysis (
Iyer et al, G3, 2016). My long-term goal is to develop computational, genomic, and functional methods to identify disease-defining and disease-modifying genes using large-scale human genomics studies and interaction studies in model systems, in order to connect genotype to phenotype in developmental disorders.
Genomic studies to dissect phenotypic heterogeneity
Previously, we proposed a paradigm for complex disease where a variably-expressive CNV sensitizes the genome for disease, but in concert with other variants elsewhere in the genome leads to a range of severe phenotypic outcomes (
Girirajan et al, NEJM, 2012). We have since continued exploring this paradigm, correlating global genomic features towards clinical outcome by assessing CNV and sequence variants in large neurodevelopmental disease cohorts. We found that the male sex bias within neurodevelopmental disorders is influenced by the presence of specific comorbidities, specific CNVs, mutational burden, and pre-existing family history of psychiatric and behavioral phenotypes (
Polyak et al, Genome Med. 2015). For example, we found that girls carried a higher burden of large CNVs compared to boys for autism or intellectual disability, but this difference diminished when severe comorbid features, such as epilepsy, were considered. In collaboration with researchers at UC Davis and Penn State, we found that individuals with autism are more likely to carry duplications compared to healthy individuals (
Girirajan et al, HMG, 2013). By analyzing longitudinal data from a special education cohort of 6.2 million children, we found that the increase in the prevalence of autism can be explained by a concomitant decrease in the prevalence of intellectual disability, suggesting diagnostic recategorization of individuals from intellectual disability to autism (
Polyak et al, AJMG, 2015).
To further study the effects of variants in the genetic background towards phenotypic variability, we use the
16p12.1 deletion as a paradigm for complex disease. We have formed an international consortium of more than 50 researchers and clinicians to recruit and generate targeted exome and whole-genome sequencing (WGS) data and detailed phenotypic data from over 400 individuals in affected families. Our results suggest that variably-expressive CNVs confer differing sensitivities to disease, and the manifestation of specific phenotypes is contingent upon the genetic background. We are currently performing WGS and quantitative phenotypic measures for a larger set of 16p12.1 families, as well as analyzing over 2,000 exomes of individuals with more than 25 rare pathogenic CNVs, to connect CNV and second-hit genes in neurodevelopmental pathways with specific phenotypes.
During our analysis of large sets of genomic data, we identified several limitations of current targeted sequencing methods. Therefore, we have developed several computational algorithms to evaluate the effectiveness of genome sequencing technologies. For example, we found that some regions of the genome are routinely under-represented in exome sequencing experiments, causing certain disease-associated genes to be missed and emphasizing the importance of WGS (
Wang et al, Sci. Rep., 2017). We developed novel metrics to identify such areas of sparse coverage in sequencing data as well as a
software package to calculate these metrics.
Functional analysis of CNV pathogenicity
Identifying the causative genes within variably-expressive CNVs and the mechanisms responsible for the observed phenotypes has been challenging, as the extensive phenotypic heterogeneity observed in individuals with these CNVs suggest the role of multiple genes and their interactions both within and outside the CNV in modulating the phenotypes. In contrast to syndromic CNVs, where the causative gene has been extensively studied using mouse models, a systematic functional evaluation of each gene within variably-expressive CNVs and its interactions requires a model system that is sensitive to genetic perturbations but, at the same time, allows for performing high-throughput functional studies in the nervous system.
Drosophila melanogaster provides such a model, as developmental processes, synaptic mechanisms, and neural structure and signaling are conserved between flies and vertebrates.
Because of this, we have established a robust
Drosophila research program in my lab. Recognizing that fly models are ideal for performing high-throughput screening, we developed a battery of highly sensitive functional assays in
Drosophila melanogaster for testing homologs of conserved neurodevelopmental genes. For example, as the
Drosophila eye is an accessible and sensitized experimental system for quantitative studies of nervous system development and function, we developed a computational method called
Flynotyper that allows us to calculate phenotypic severity due to gene disruption in a high-throughput manner (
Iyer et al, G3, 2016). For deeper cellular phenotyping of the developing fly eye, we are also using confocal microscopy techniques to examine changes in cellular structure and molecular mechanisms caused by gene knockdown. Overall, we are performing detailed phenotyping for dosage sensitivity of >150 fly lines of genes in eight CNV regions, and testing pairwise interactions of these homologs in order to identify common mechanisms of disease pathogenicity--thus developing the first models for CNV pathogenicity in flies. Our work emphasizes the need for a function-based analysis in addition to sequencing studies towards discovery of gene function in neurodevelopment.
My future research will use integrative strategies that combine data from large-scale genomic studies and multiple model systems using novel molecular and computational methods. With these approaches, we will study hundreds of genes and genetic interactions in a high-throughput manner to dissect the complexity of genetic disease, untangle molecular subtypes of disease, and provide an understanding of disease pathogenesis based on mechanism. I envision that my research will further transition towards molecular neuroscience, developmental biology and biochemistry, as we continue to uncover the deeper complexity of gene function in neurodevelopment.